Baynote is one of a number of recommendation technology providers which licenses its product to commercial companies. As we’ll see in this article, Baynote places particular emphasis on real-time user behaviors – which Baynote claims goes beyond Amazon’s “first generation” approach to recommendations. One thing that we’ve discovered so far in our series on recommendation engines is that every company in this market – including those which create their own platform, like Amazon and Netflix – have differing approaches and ideas on what makes a good recommendation engine. We spoke to Baynote founder and CEO Jack Jia, to find out why he believes their approach trumps Amazon.
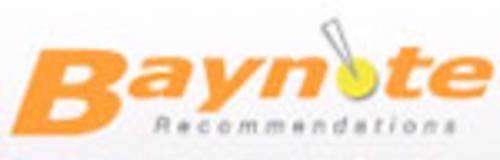
Baynote’s focus is to analyze the current behavior of a community to come up with recommendations – it purposely puts less emphasis on past user actions such as page views and purchase history.
In positioning his company Baynote against the “king of recommendations” Amazon (as we’ve termed them), Jia told us that Amazon is a first generation recommendation engine because it still largely relies on collaborative filtering. The gist of Jia’s issue with collaborative filtering seemed to be that it focuses too much on analyzing past user data – it doesn’t account enough for real-time user behavior, which is what Baynote does.
As Jia described it to us, Baynote observes real-time user behavior on a site and looks for implicit, emergent patterns. It uses collective intelligence and an affinity engine to analyze the data. Common behaviors which it tracks include page refers, queries, mouse movement, time spent on a page, peer behavior (see note about communities below).
The notable exceptions, which Baynote doesn’t account for as much as other recommendation engines, are page views and purchases. Jia told us that Baynote considers those two things “not very useful in recommending products or content” because they can lack contextual relevance and aren’t necessarily real-time. User engagement, he said, is the true value of measuring what content is useful.
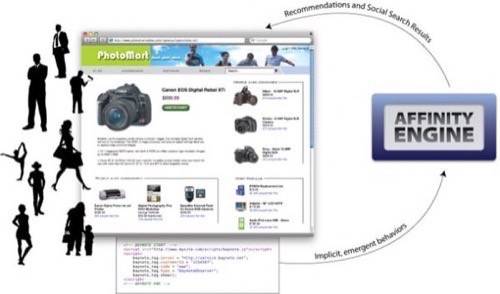
A note on how Baynote defines communities. Basically the system splits users into various communities and sub-communities. Let’s take the example of cameras. As Jack Jia explained, cameras can be split into many categories and sub-categories. There may be a community of Nikon cameras, and within that a sub-community of ‘high-end’ Nikon cameras, and so on.
What Makes Baynote’s Personalization Different to Amazon’s?
It’s obvious that Baynote has some complex technology under its hood. That includes a concept that Baynote calls UseRank – obviously named after Google’s famous PageRank, but Baynote’s UseRank is focused on user behavior on a website (rather than links). Baynote has patents pending on this technology, which it uses to achieve “real-time contextual personalization”. But what makes this form of personalization better than Amazon’s?
Jack Jia told ReadWriteWeb that the “personalized profile approach”, which the likes of Amazon use, isn’t something Baynote puts emphasis on. Baynote’s position is that traditional personalization doesn’t work, because those approaches don’t take into account user intent and context. A classic example is one that we outlined in our post 5 Problems of Recommender Systems: just because you bought something yesterday, doesn’t mean you want to buy a similar thing today (e.g. I might buy a birthday present for my sister one day, and a new CD for myself the next). This is why Baynote feels the need to focus on real-time intent and context. As an example Jia showed us how one of their customers uses Baynote technology to recommend items to users.
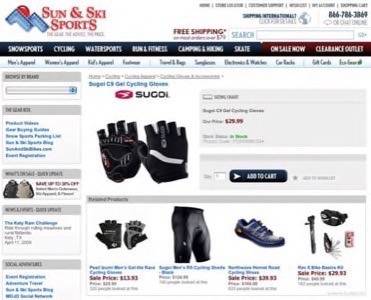
UPDATE: the below paragraph doesn’t refer to Sun & Ski, but another Baynote customer that Baynote showed us at the time of the interview. Baynote has subsequently requested that the original company be removed from the post. We’ve left the text as-is, however it doesn’t refer to Sun & Ski.
In the example, users are shown a “you may also like” section to the right. We asked how this was different to Amazon’s “Customers Who Viewed [or Bought] This Item Also Viewed” recommendations? Also we wondered if there might be a bias in Baynote’s top recommendation, as most users would naturally click the top item first. To the latter point, Jack Jai reminded us that Baynote disregards page views. He further explained that Baynote measures actions and engagement on pages – so for example if a user clicks on the top link, but then clicks the back button straight away, that would be counted as a negative user measurement in its recommendation engine. But if the user scrolls on the page, highlights things, etc, then it’s a positive measurement. Baynote is not just tracking clicks, but engagement on the page. It says this this approach has led to increases in sales for [above customer] and other retail clients.
Conclusion: Don’t Count Personalization Out Just Yet
Baynote has about 200 eCommerce, media and enterprise clients – half of those are in the B2B sector. So it’s not just the retail sector in which recommendations can be deployed. For example, Baynote told us about the use of its recommendation technology on Motorola’s intranet – where the system observes employee activities, so that it can recommend content and improve the search experience.
Baynote is also an example of a Software-as-a-Service. It can be easily implemented, is on-demand and Baynote claims that it works with any site and search engine.
Overall, we were impressed with Baynote’s recommendations system. However, we’re not necessarily convinced that it trumps the personalization approaches used by the likes of Amazon and richrelevance (founded by an ex-Amazon executive). We’re certainly not ready to write off Amazon’s recommendations as mere “first generation” technology quite so quickly as Baynote. Further, we think that up-and-coming companies such as richrelevance, which we will profile shortly in this series on recommendation engines, have interesting innovations in the area of personalized recommendations.
So, there’s room for many approaches – although we hold Baynote’s technology in high regard, based on what we learned about it. Tell us your thoughts on Baynote’s approach in the comments below.