There’s a public relations brochure template someplace that reads, “________ is changing the way the world does business.” If this were a Mad-Lib, you could insert the proper noun of your choice. Historically, evolutionary changes in both business and the economy that supports it, have mandated the need for subsequent changes in technology. There are certain very notable exceptions (thank you, Tim Cook), but let’s be honest and admit that databases didn’t spring up from gardens like daisies and change the landscape of business from winter into spring. There was a need for relational databases that went far beyond keeping up with the competition.
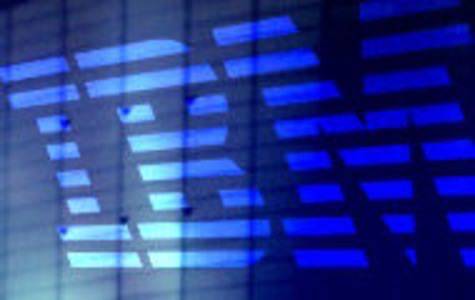
So when companies say that big data will change the way you work… really? Is that the best value proposition that vendors can come up with – “It’s coming like a thunderstorm, so you’d better be prepared?” In the final part of ReadWriteWeb’s conversation with IBM Vice President for Big Data Anjul Bhambhri, which continues from part 2, I told her a true story about a customer on a vendor webcast that was set in its ways and resisted the change that the PR folks were saying was inevitable.
Scott Fulton, ReadWriteWeb As you probably know on a deeper level than I, the reason for database siloing dates way, way back to the 1970s and ’80s when computing products were purchased on a department-by-department basis. Way back in the mainframe era – which IBM helped the world inaugurate (so it’s your fault) – computing products were purchased, deployed, configured, programmed by the people in finance, in budgeting, in human resources, in insurance management, in payroll. And these were all disparate systems. The archival data that has amassed from this era is based on these ancient foundations, which it only seems to make sense to those who developed software for a living to say, “We’ve got the power to make it all fit together now, why not use it?”
But I was on a webcast the other day listening to a fellow for about 60 minutes, making exactly your case. Why we should remove silos from big organizations, and make the effort to develop ways of merging big data into “usable meshes,” he called them. It was a good point and it lasted for 60 minutes. And the first question he got from somebody texting in was, “Simple question: Why?” And the presenter said, “What do you mean, why?” And he said, “Okay, don’t you know that these silos exist for a reason? Businesses like ours [I think he was in banking] have departments, and these departments have controls and policies that prevent information from being visible to people in other departments of the business.” And he asked, “Why would you make me spend millions of dollars rearchitecting my data to become all one base, and then spend millions more dollars implementing policies and protections to re-institute the controls that I already have?” And the presenter was baffled; he never expected that question, and he never really answered.
So I wonder if that question has ever been shot your direction, and you ever batted it out of the park?
Anjul Bhambhri, IBM: What you said, I agree with that completely. There’s a reason this has happened. And it doesn’t matter what we do; you can’t just get all this data into one place. Data is going to be where it is in an enterprise. There may be department-level decisions that were made, department-level applications that are running on top of it. And nobody’s going to like [some guy coming in saying] “Let me bring this all together.” It’s too much of an investment that has been made over the years. In hindsight, we can always say this is the way things should have been architected. But the reality is that this is how things have been architected, and you run into this in almost all the enterprises.
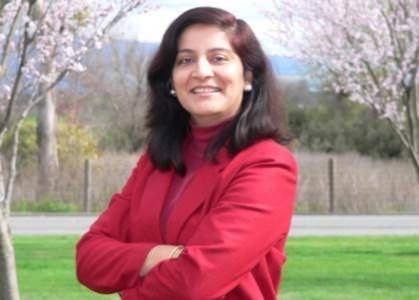
If they start ingesting data from these sources, they have to be cognizant of the fact that they could be dealing with very large volumes. So they don’t want the whole IT infrastructure to collapse because they didn’t anticipate what hardware they should have in place.
Anjul Bhambhri, VP for Big Data, IBM
My response and suggestion – and we’ve actually done it with clients – has been that, you leave the data where it is. You’re not going to start moving that around. You’re not going to break those applications. You’re not going to just rewrite those applications… just to solve this problem. Really, data federation and information integration is the way to go. Data is going to reside where it is. IBM has done a very good job in terms of our federation technology and our information integration capability, where we are able to federate the queries, we can pull the right set of information from the right repositories wherever it lies. Then we can obviously do joins across these things so that we can do lookups of information in maybe the warehouse, and we can correlate it with information that may be coming from a totally different application. And all of this is done while preserving the privacy and security, the accessibility, the role-based policies that may have been implemented. We can’t ask people to change all that. We can’t have departments just start changing it. If there’s some data that they don’t want another department to see, then that has to be respected.
Even in the big data space, you can imagine this is a question which comes down a lot from the big enterprises that have made huge investments in these technologies. They’re not going to have one data repository. It’s all a heterogenous environment, and it’s going to continue to stay that way. That is not going to change, nor do we expect it to change.
Also, you don’t really want it to change, right? People have built those repositories and those applications because they were the best choices at the time for that class of applications. Or they may have bought solutions from vendors like SAP, or they could be ERP or CRM systems that they have bought from various vendors. Those cannot all be thrown away. If the companies were using CRM applications, for example, to really understand aspects of the customer, now we want you to continue to use that, you don’t stop using that application. But you may need to augment the information that you can get from a CRM application with what maybe social media offers around the customer, so you can really get more like a 360 view of the customer. Don’t abandon what you’ve got, but integrate. Be able to bring in these new data sources, and the level of tooling [necessary] to be able, in that single dashboard, to pool the information from these CRM applications [and] from these new data sources that may be Facebook or Twitter or text messages – to correlate this information and maybe show aspects of the customer where, if you were only looking at the CRM application, would be incomplete.
I really think federation and integration is the way to go here, and not dictate that data be moved or be in a single repository. Heterogeneity is a reality, and we have to accept it and provide the technology that actually takes advantage of that heterogeneity, and respect the decisions that the customers have made.
Scott Fulton: You believe the emerging tools that we talked about earlier, that we will need the data scientists to effectively learn how to use, will be tools that won’t change the underlying foundation of data as we currently have it, but simply add a layer of federation on top of that?
Anjul Bhambhri: What is happening behind the scenes – To the data scientists, we really just want them to focus on that. Their expertise is needed with other data sources that are important to the organization. Given their subject matter or domain expertise, they are the best ones to recommend where else is information needs to be gleaned from. And then of course, the IT group has to make sure those can be dictated, plotted, in the data platform. They cannot say, “Okay, we have two applications running on the mainframe and all these silos, but we can’t bring in more data sources.” They obviously have to facilitate that.
But from a tooling standpoint, the data scientist should be able to really – the tools have to be so easy that they can say, “If I want to know this about customer X,” and if I ask, “Just pull all data available on this customer,” that could be information coming from the warehouse, from the CRM application, from the transactional system with the latest set of transactions that the customer has had in the last day, month, whatever. And if there’s a way to say, “Okay, what is the last interaction that we had with this customer?” Maybe the customer called in, maybe he went into our Web site and did some online stuff. It could just be, random pieces of unrelated information about our customer, or it could be aggregated around the customer. But they should be able to look at, visualize these things in the tool. Because you can imagine that, just random text about this customer also has to be presented properly, so that based on the questions that are being asked, maybe things have to be highlighted, annotated so it’s visually pretty clear to the data scientist how the person exploring this data, that they don’t miss out on some important aspect of it.
Making data bigger and more consumable
Anjul Bhambhri, VP for Big Data, IBM: I think the tools have to be very sophisticated, that they take away anything that has to do with the underlying technology, so their federation happens behind the scenes. How many repositories were queried to pull this information out? What were the seven different data sources that were brought in? All of that has to be just completely hidden.
That’s really the direction we are moving in, and for any vendor to really help our customers to embrace what’s happening in this Internet era, and really understand aspects of the business they are in, I think it’s pretty critical that this happens. We’ve seen, people have been collecting data from sensors forever. More and more things are getting instrumented, so there’s more sensor data, but it’s not like there was no sensor data a few years ago. But they just never knew how to analyze this data quickly. There were no tools available to do that. So now… at least we are starting to see, and put in front of those customers, here are the possibilities, here’s how the data can be analyzed. If there’s a lot of noise in the data, we can filter it out. So I think that is what is going to make data go to the next level; it’s going to be all around consumability.
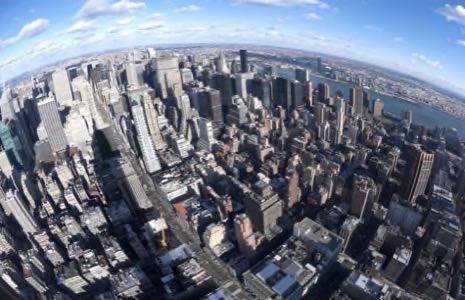
Scott Fulton, ReadWriteWeb: As complicated as these tools will need to be, is it fair to just proclaim today, right now, that they will have to be delivered as a service, as a cloud-based application, rather than as software as we have come to define it since the 1980s?
Anjul Bhambhri: I would say both. It makes sense for some of these capabilities to be available as a service. Just like on Yelp, you go look at reviews of restaurants, if there was something you wanted to know about it – “How is my XYZ plan being perceived in a particular geography?” – and there was a service that could provide that information, underneath they would still be using these big data platforms and capabilities, but consumers would certainly look at the value of the service like that. I think analytics being available as a service is going to show up more and more.
If they start ingesting data from these sources, they have to be cognizant of the fact that they could be dealing with very large volumes. So they don’t want the whole IT infrastructure to collapse because they didn’t anticipate what hardware they should have in place.
Anjul Bhambhri, VP for Big Data, IBM
These could be public services. There could be department-level services or private clouds, like we are seeing emerge. Instead of saying, “Here’s all the data,” they may say, “What are the kinds of questions you need to ask? What information do you need, and we will provide that as a service.” They can then still maintain control of what they want to maintain control of. Within the companies, being able to provide some of this information as a service, underneath they may still be using the big data kind of technologies, but externally to the consumers within the enterprise, it will be available as a service. Anybody who wants to implement this as a service, somebody has to be using these tools and these technologies to build those services.
Scott Fulton: Earlier, you characterized the data scientist’s role and distinguished that from the IT manager’s role. You mentioned that the data scientist needs to be someone focused on the meaning of how the data relates to each other, and giving the instructions to the IT manager who would be processing the data and keeping the warehouse. I take it that, by that characterization, you intentionally mean to distinguish that data scientist as someone who is outside the IT department, am I right?
Anjul Bhambhri: Certainly in most of the big enterprise customers, that’s what we see. But it depends. If it’s a smaller setup, I could see those roles getting merged together. But yes, for the most part, I would make that distinction. The data scientist is somebody who really observes and discovers the data, and who is really focused on the data aspect, and what the data is telling them. And then the IT department is building that infrastructure to make sure the data platform, even if they have five warehouses, is not just limited to structured data – that they can bring in their ideal data sources, and are building an infrastructure that is scalable, that they can handle these large volumes of data that might be coming in. If they start ingesting data from these sources, they have to be cognizant of the fact that they could be dealing with very large volumes. So they don’t want the whole IT infrastructure to collapse because they didn’t anticipate what hardware they should have in place. They have to think about all of that infrastructure, at IT. Whereas the data scientist should not have to worry about that, right? Their core competency and focus should really be in gleaning that information and the value that can be derived from it.
Yes, for the most part, I would say there’s a separation. But in smaller setups, it’s possible that they don’t have the luxury to do that. People have to play multiple roles and wear multiple hats.
Stock photo by Shutterstock